Unlocking the Power of Data Science in Social Housing
In the ever-evolving landscape of social housing, the role of data science has become increasingly pivotal. Mobysoft’s recent (Not So) Weird Science: Critical Considerations for Success When Using Data Science to Improve Tenant Experience webinar saw Mobysoft head of product, Natalie Tuer, and senior data scientist, Jack Pawson, explore the significance of data science in this sector, emphasising its potential to help organisations interpret data from various systems, overcome challenges posed by unstructured data, and provide valuable insights for strategic decision-making. This blog post delves into the crucial aspects discussed and demonstrates how data science can revolutionise social housing management approaches by utilising predictive analytics and data engineering.
Interpreting Data from Multiple Systems
Social housing providers often deal with a myriad of data, coming from diverse sources. This data includes information about repairs, payment types, and payment frequencies. To make sense of this deluge of data, data science comes to the rescue. It helps organisations interpret data from these disparate systems, facilitating the identification of patterns and trends. For instance, predictive analytics can be employed to predict when equipment or facilities within a property are likely to fail, leading to proactive maintenance. This not only saves on operational costs but also enhances tenant satisfaction by preventing inconvenient disruptions. By analysing payment data, predictive models can also assist in optimising rental rates, ensuring that properties are priced competitively while maximising income and minimising vacancies.
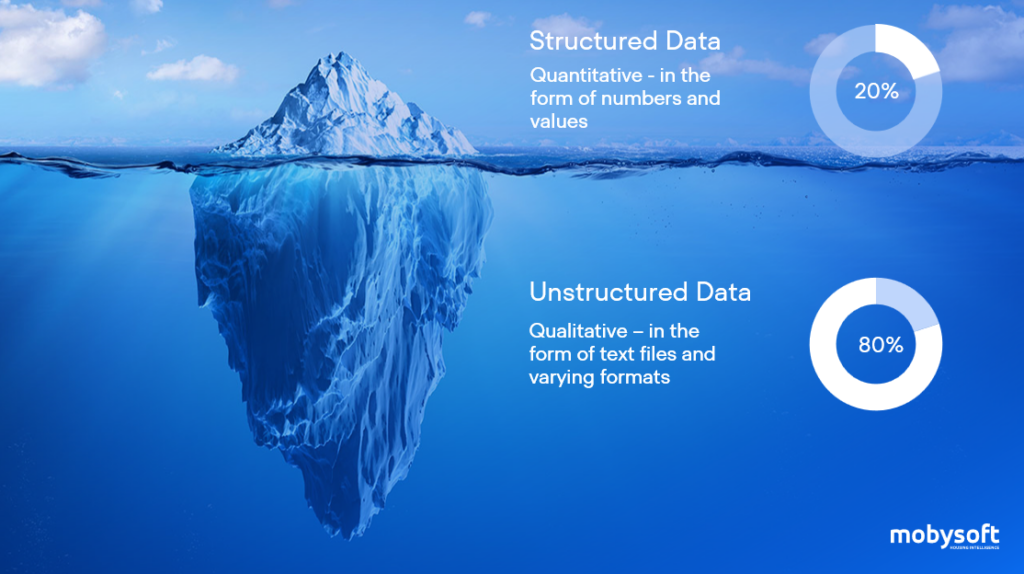
Overcoming Challenges of Unstructured Data
Data in the property management sector is not always neatly organised or defined according to set criteria. Put simply, unstructured data is data (typically large collections of files) that isn’t stored in a structured database format. Unstructured data has an internal structure but is not predefined via data models. Such data could be human, or machine generated in a textual or a non-textual format. This type of data lacks predefined criteria and can be challenging to analyse. However, data science is adept at handling unstructured data. Machine learning and natural language processing techniques can be employed to extract meaningful information from these sources. For example, by analysing reports on repairs, data scientists can identify recurring issues and trends in maintenance requests, allowing property management companies to proactively address common problems.
Presenting Relevant Information for Strategic Decision-Making
The ultimate goal of data science in social housing management is to provide accurate, relevant information that can be used to formulate strategic approaches to income functions and repairs and maintenance services. By consolidating data from various sources into a central data repository, social landlords can gain a comprehensive view of their operations. Data engineers play a crucial role in this process by integrating data sources, cleaning and transforming data, ensuring scalability and performance, and addressing data security and compliance.
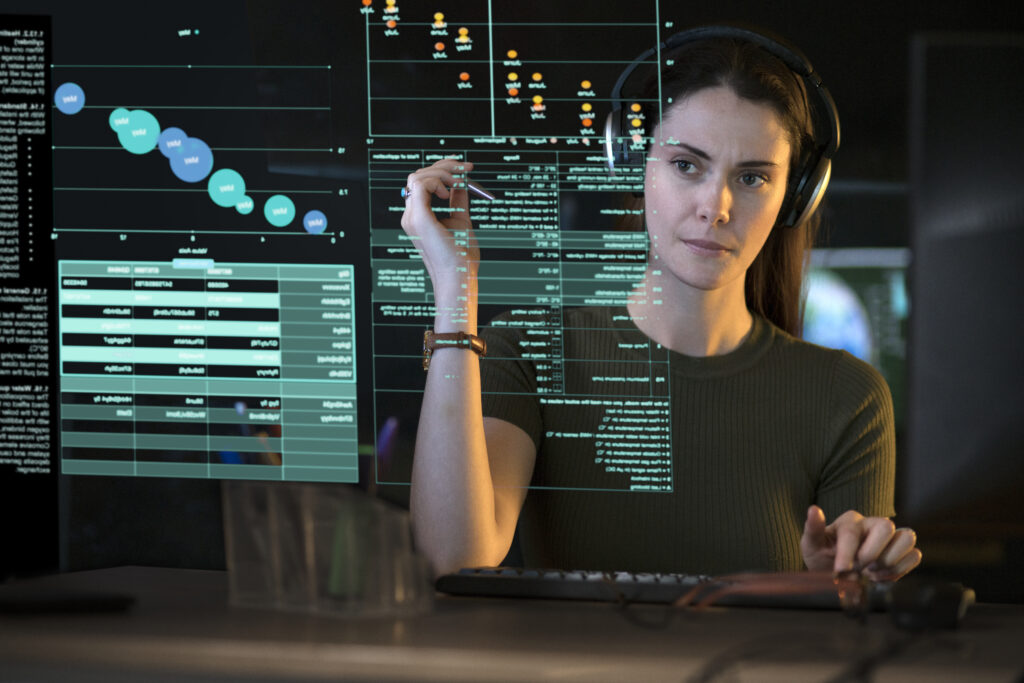
Utilising Supervised Machine Learning
Supervised machine learning is a powerful tool in data science, especially for social housing providers. The practice involves analysing large datasets to create labelled data (data that is annotated with meaningful tags, or labels, that classify the data’s elements or outcomes). This labelled data allows users to surface the right data streams, analyse them, and allocate resources according to the actionable insights that arise. For instance, supervised machine learning can be used to analyse tenant data and identify characteristics or behaviours that correlate with tenant turnover. Armed with this knowledge, social landlords can implement strategies to improve tenant retention, ultimately leading to increased profitability and tenant satisfaction.
As we’ve explored here, data science can prove to be a powerful ally to social housing providers. By offering advanced tools to interpret data, overcome unstructured data challenges, and present valuable insights, data science is transforming the property and repairs management approaches within the social housing sector. In proactively leveraging predictive analytics, data engineering, and supervised machine learning, social housing providers are beginning to optimise their operations, enhance tenant experiences, and make data-driven decisions that lead to greater efficiency and profitability. As the social housing sector’s operating environment continues to evolve (often into one laden with more and more challenges), it’s increasingly evident that embracing data science is not just an option; it’s a strategic imperative.
If you found this article interesting then you might want to check out our forthcoming Rise of The Machines: How Supervised Machine Learning Will Solve the Damp & Mould Crisis webinar on Wednesday 15 November. Head over to our dedicated event page to register your interest today.
- Seasons Greetings from Mobysoft! - December 24, 2024
- Spotting the Silence: The Crucial Role of RepairSense in Social Housing Maintenance - November 19, 2024
- How Data-Driven Insights Can Improve Tenant Satisfaction in Temporary Accommodation - October 18, 2024